EViews 14 New Econometrics: Estimation and Forecasting
EViews 14 includes a number of new estimation techniques:
- Facebook™ Prophet
- Quantile ARDL Estimation
- ARDL Improvements
- MIDAS GARCH Estimation
- Elastic Net Enhancements
- Improved Lasso Selection Models
- Local Projection Impulse Response (LPIRF) Analysis
- Bootstrapped Structural VAR and Structural Variance Decomposition Confidence Intervals
- Improvements to Bayesian Time-Varying Coefficient VAR Impulse Responses
Facebook™ Prophet
Prophet is an open-source forecasting tool developed by Facebook™ with both Python and R interfaces.
EViews 14 introduces an easy-to-use graphical user interface to Prophet to allow users to harness the power of Prophet without needing to worry about the complexities of Python or R.
Quantile ARDL Estimation
The Quantile Autoregressive Distributed Lag (QARDL) model, introduced by Cho, Kim, and Shin (2015), is an extension of traditional ARDL models to capture the dynamics of conditional quantiles (percentiles) of the dependent variable. While conventional models provide insights into the mean responses of the dependent variable to changes in predictors, QARDL models allow you to model the effects of changes in predictors on the quantiles of the dependent variable.
While QARDL models may be estimated using generic quantile regression tools by specifying models using with the appropriate levels, lags and lag differences of variables, EViews 14 offers an easy-to-use native interface for estimating QARDL and QNARDL models
ARDL Improvements
EViews 14 expands the calculation of heteroskedasticity and autocorrelation consistent (HAC) estimates of coefficient covariances in ARDL equations. Previously, HAC coefficient covariances were only computed for the base ARDL estimates of the Intertemporal Dynamics (ITD) representation of the specification. HAC estimates were not available for the Conditional Error Correction (CEC) or Error Correction (EC) forms of the model.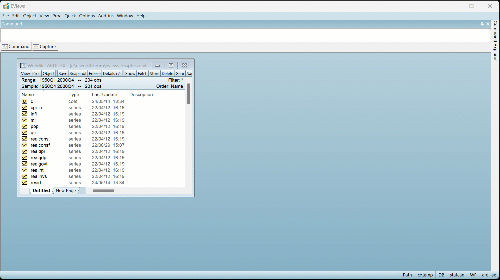
MIDAS GARCH Estimation
Mixed Data Sampling (MIDAS) regression is an estimation technique which allows for data sampled at different frequencies to be used in the same regression. For MIDAS GARCH models, the approach is to incorporate information from a large number of lags of a lower frequency series into the variance specification of an ARCH regression; incorporating, for example, lags of quarterly data in a monthly data GARCH model. One of the main advantages of this approach is that it allows decomposition of the variance into short and long run variances.EViews 14 estimates the multiplicative component MIDAS GARCH(1,1) model of Conrad and Kleen (2020).
Elastic Net Enhancements
While prior versions of EViews did offer tools for elastic net estimation, EViews 14 completely updates the existing features to offer more efficient algorithms for estimation, added control over coefficient penalties including individual coefficient weights and coefficient bounds, more efficient cross-validation tools for selecting models along the lambda path, and enhanced views and tools for examining the behavior of coefficients, estimation objectives, and model fit statistics along the path.
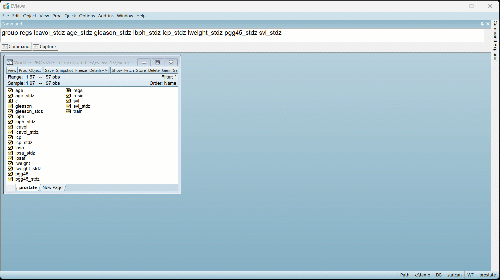
Improved Lasso Selection Models
In addition to the elastic net enhancements, EViews 14 offers the same improvements for the lasso-selection estimation method, allowing greater control over the selection procedure, and an improved suite of post-selection diagnostics.
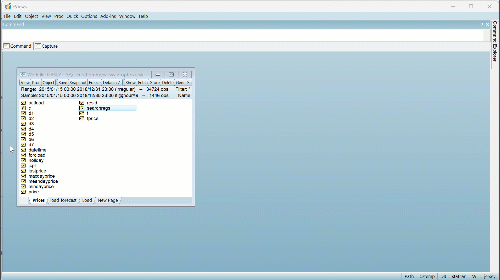
Local Projection Impulse Response (LPIRF) Analysis
EViews 14 supports estimation of impulse responses via Local Projection (Jordà 2005) using both standard sequential and and joint estimation. For sequential estimation, you may handle the serial correlation effect on covariance estimates using non-parametric HAC corrections.
Bootstrapped Structural VAR Confidence Intervals
EViews 14 can now perform monte carlo and bootstrap simulation for both SVAR impulse response and variance decomposition.
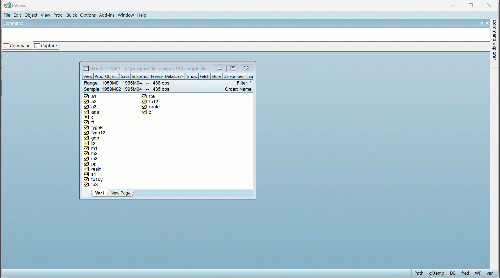
Improvements to Bayesian Time-Varying Coefficient VAR Impulse Responses
EViews 14 offers new tools for producing BTVCVAR IRFs at fixed horizons, allowing you to examine how the system response at a given elapsed time has changed with changes in the impulse date.
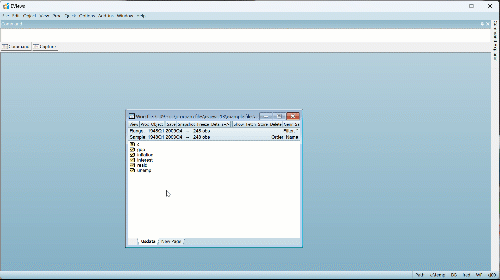